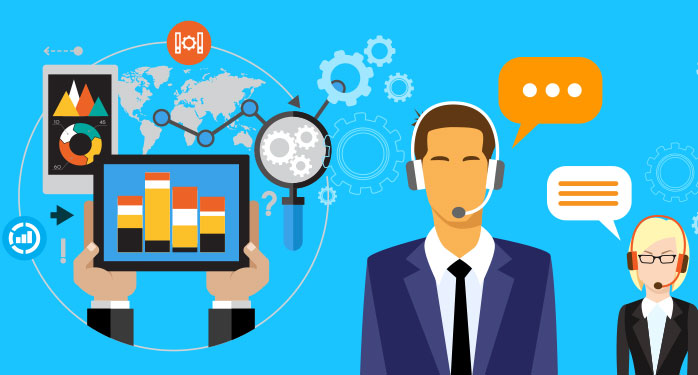
Objectivity
Contact center reliability in terms of delivering the value promised to customers depends largely on the performance of individual employees. Thus, in order to ensure that optimal effort is given on each and every call, management teams are required to implement contact center quality monitoring (QM). In most cases, this task is performed by a dedicated QM staff whose goal it is to ensure that required procedures are met while also conveying the messages and tones unique to each client. While QM focuses on assessing and improving an employee’s individual skills, its general purpose is two-fold: to meet the performance demands of contact center clients while also ensuring that operations remain compliant with industry standards.
Manual Monitoring – An Inherently Challenging Process
Typically, the QM process at most call centers involves an individual agent (be it a manager, supervisor or dedicated QM analyst) listening to individual calls and grading out an employee’s performance. After each assessment the results must then be shared through the appropriate channels, beginning with supervisors or shift managers and then with the individual employees themselves. These individual one-on-one sessions between employee and analyst or supervisor and analyst are essential in order to identify areas that need improvement. Finally, follow-up evaluations are required in order to ensure that education has been effective at helping employees improve the customer experience on his or her calls.
While QM efforts are vital for improving contact center performance, the aforementioned process carries with it a number of inherent inefficiencies. These include:
-
Resource management:
Managing QM can force executive teams to walk a tight line between meeting the demands of client output and improving processes and performance. When developing a QM strategy, many often first look to supervisors and managers to perform these tasks. While their familiarity with the skills and weaknesses of their individual team members may offer valuable information to this process, adding QM to their already full schedules often requires asking them to sacrifice time and effort being dedicated to other areas. Oddly enough, the net impact of having supervisors perform QM is often a decrease in overall performance due to less attention being paid to other vital areas of operation.
The next solution would then seem to be to create a dedicated QM staff whose workflow would be centered on call evaluations and employee training. This eliminates the need for management to be extensively involved in the QM process and creates a team of subject matter experts that employees may be able to rely upon as a resource. However, it is important to keep in mind that creating such a resource would likely require pulling employees that have demonstrated the highest levels of performance off of the phones in order to effectively perform this function. While the anticipated payoff is that they would then be able to convey their skills on to other employees, the question becomes how long the center would be able to wait for the rest of its staff to replace the newly named QM analysts’ levels of performance?This leads to the final concern that manual QM presents in regards to resource management: time. If a team of supervisors or QM analysts is expected to turn the trends discovered during call evaluations into actionable information that helps employees improve customer engagement, then they need the time to both listen to calls and provide education. While the expectation is already in place, that call monitoring will be a primary function of a QM team, the amount of time needed to follow up with employees and managers if often not accounted for. Once evaluations have been done, the QM team must then pull supervisors away from their regular tasks to share results, and then pull employees off the phone to provide education. For contact centers already straining to deliver optimal output levels, such allocations of time may prove to be too great of a cost.
-
Limited sample sizes:
Because the QM process can be so expensive and does require so much time, managers or analysts can only afford to perform their assessments using small sample sizes. In many cases, the actual rate of assessed calls may often represent less than one percent of a contact center’s total output. One has to wonder if such a limited volume is able to produce an accurate depiction of overall employee performance.
Employees may argue that judging their capabilities on the phone off of a small random sample of their calls puts them at an inherent disadvantage. Depending upon their specific roles and responsibilities, they may feel more comfortable in certain areas than in others. Thus, while evaluating a call for a client or product with whom they are unfamiliar may serve to highlight their weaknesses, it fails to identify those areas which they may view as their strengths.Along with offering a potentially unbalanced assessment of an employee’s skills (or lack thereof), random call monitoring also may fail to show if employee education has truly taken hold. For example, if an employee were marked for a reassessment after having received training and education, reviewing a call from the week following said training may not show what he or she has learned and implemented than one from two-three weeks later. The trouble is, when pulling random call samples, QM analysts often have little control over the time frames from which their calls come from.
One might argue that the solution to the problem of limited sample sizes is simply to increase his or her QM staff. However, wouldn’t such action simply serve as an example of throwing more resources at a process that’s already been proven to be somewhat ineffective?
-
Objectivity:
The final challenge that comes with manual QM monitoring is delivering objective results. When preparing the criteria that will be used to assess employee performance, the question has to be asked as to the basis used to develop it. Is it being prepared by upper-level executives based solely off of perceived client expectations, or former front-line employees thinking only of what callers may want to hear? Some may think that the best way to create call standards is to combine the expectations of both sides. The problem is that often produces evaluation categories that are so multidimensional that they become inadvertently vague.
Consider this example: For an evaluation of “Customer Service,” the evaluation criteria may be:
- Resolves customer doubts as well as future concerns
- Engages customer in discussion rather than simply reading scripts
- Maintains a proper tone at all times
- Keeps call focused on product and service aspects
- Expresses sincere appreciation for customer loyalty
- Maintains professionalism throughout the call
This criteria presents two problems: first, certain points seem to be contradictory to each other. How is an employee to engage a customer in conversation while still keeping a call solely focused on product and service aspects (information typically found only in call scripts)? Or how sincere does thanking the customer for his or her loyalty seem if his or her call was due to doubts and concerns about the brand’s products? And how exactly can one judge an employee’s abilities to resolve future concerns?
Second, expressions such as “proper tone”, “professionalism” and “sincere” are often open to interpretation. What a QM analyst or supervisor may view as cold and unemotional may be what an employee believes to be forthright and professional. When grading employee performance in these areas, how are such contradictions and ambiguities judged? Could a single employee action produce a high assessment score in a certain area along with a lower mark in another?
Finally, one has to consider the discretionary powers of the analyst or manager performing the assessment. What external factors may be influencing their evaluations? Empathy, emotion and even professional or personal relationships may come into play when judging the performance of another. An example may be to compare two employees, one who has a strong evaluation history while the other has struggled. In one case, the evaluator may judge the former more harshly based upon increased expectations, while the latter is scored more favorably simply based off of incremental improvements. Ultimately, do such assessments really paint a clear picture of actual performance?
The Solution is Speech Analytics
The solution to solving the problems inherent with manual QM is truly revolutionary, in that it involves completely altering the employee assessment and reporting source. Implementing speech analytics into QM eliminates all of the shortcomings of manual processes, adding value through both process improvements and resources saved. How so? Consider the obstacles to effective manual monitoring mentioned above, and how speech analytics helps to address them:
-
Assessment objectivity:
Rather than relying on vague, multidimensional evaluation categories that leave room for interpretation, analytics programs break down assessment criteria into well-defined agent skills. For example, for the aforementioned evaluation element of “Keeps call focused on product and service aspects,” an analytics evaluation will create a set of definable criteria that meet the standard of “focused.” These may be “Did the employee mention service aspect X?” or “Did the employee refer the caller to Section X of the owners’ manual?” If, in addressing a callers questions about the product or service in question, the agent touched upon these pre-defined points, he or she meets the assessment criteria. Gone from the equation is the emphasis placed on ambiguous speech patterns and behavior.
With these pre-defined skills already identified, the analytics system can then continuously compare them with consistent employee performance. If and when key skills begin to be omitted from conversations with callers on a consistent basis, the system notifies both the employee and his or her respective supervisor or manager.How effective has this proven to be compared to manual processes? Comparisons between contact centers implementing both methods have consistently shown the analytical systems to produce assessment results lower than those generated through traditional methods (in most cases, significantly). These studies serve to show just how impactful objectivity can be to the QM process by highlighting how, in many cases, manual evaluations could actually hinder performance rather than look for ways to improve it.
-
Sample volumes:
Where QM analysts or managers can only afford to review a limited sample of calls, analytical systems measure and review them all. Thus, a comprehensive view of each employee’s performance is made available, not just a simple snapshot of it. With every pre-defined skill reviewed in each call, QM analysts do not have to worry about any issues falling through the cracks.
Not only does this allow management teams to view their employees’ call evaluations confidently knowing that they are representative of their true behavior, it also allows them to re-introduce a concept that’s often lost in the QM process: recognition. Oftentimes, employees simply view such evaluations as opportunities for higher-ups to look over their shoulders and critique their work. The byproducts of such a view are typically resentment, disengagement and low morale. Implementing an analytics system can actually help to reinvigorate stagnant operations through an emphasis on improved performance.How is this possible, especially when comparison studies have shown evaluation scores to go down across the board when using an analytical evaluator? It’s because it serves to level the playing field between employees, producing tangible unbiased results that don’t penalize employees for being strong in certain areas and weak in others. QM analysts don’t have to worry about the perception that certain individuals are singled out for poor performance due to the fact that the system notifies employees confidentially. They are then allowed to work with groups of employees to help work towards shared goals.
-
Allocation of resources:
With the analytical system taking the tasks of assessing and scoring calls out of the hands of QM analysts and/or supervisors and managers, it frees those employees up to focus on improvement initiatives. Given the specificity that the analytical system offers regarding exactly what performance aspects need improvement, management teams can better allocate resources to shore up areas of concern.
Conclusion
Speech analytics truly represents a revolution in the field of quality monitoring for contact centers. It improves upon all areas of manual monitoring, particularly those that carry with them inherent deficiencies. Analytics evaluators review all calls against a well-defined set of employee skills, producing unbiased actionable information that management and QM teams can then use to identify opportunities where overall performance can be improved to produce higher customer satisfaction rates and significant as well as near-immediate revenue increases.