Unveiling Data Hallucinations: When Numbers Mislead
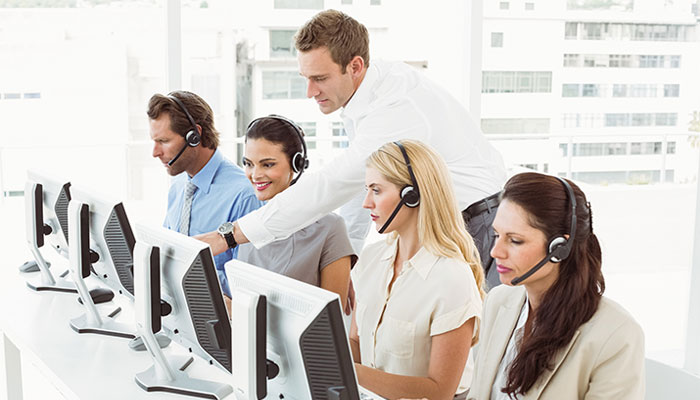
In the era of big data, numbers wield immense power, guiding decisions, shaping policies, and driving innovation. However, beneath their seemingly objective facade, lies a phenomenon known as “data hallucinations” – the distortions and illusions that can arise from misinterpretation, bias, or flawed analysis of data.
As we navigate an increasingly data-driven landscape, it’s crucial to unveil and understand these hallucinations, lest they lead us astray.
Understanding Data Hallucinations
Data hallucinations occur when flawed assumptions, biases, or errors lead to misleading interpretations of data. They can manifest in various forms, each with its own nuances and implications:
1. Confirmation Bias
This cognitive trap occurs when analysts selectively interpret data to confirm their preconceived beliefs or hypotheses, ignoring contradictory evidence that challenges their assumptions.
2. Overfitting
In machine learning and statistical modeling, overfitting happens when a model captures noise or idiosyncrasies in the training data rather than the underlying patterns, resulting in poor generalization to new, unseen data.
3. Data Dredging
Also known as “p-hacking,” data dredging involves mining data for patterns without a specific hypothesis in mind, leading to false discoveries due to chance or random fluctuations in the data.
4. Sampling Bias
When the sample used for analysis does not truly represent the population, conclusions drawn from the data may be skewed, failing to capture the full picture.
5. Misleading Visualizations
Visual representations of data, such as graphs and charts, can inadvertently distort the truth if not designed and interpreted carefully, potentially leading to erroneous conclusions.
What are the Implications of Data Hallucinations?
The consequences of data hallucinations can be profound and far-reaching, rippling through organizations, industries, and societies:
1. Misinformed Decisions
Organizations may make flawed decisions based on distorted data, leading to wasted resources, missed opportunities, or even detrimental outcomes that could have been avoided with accurate insights.
2. Erosion of Trust
When data-driven analyses produce inconsistent or misleading results, trust in data and decision-making processes can diminish, leading to undermining credibility and accountability within organizations and among stakeholders.
3. Reinforcement of Biases
Data hallucinations can reinforce existing biases and perpetuate inequalities and injustices in society, particularly when algorithms and decision-making systems are trained on biased or incomplete data.
4. Opportunity Costs
Chasing false signals or acting on erroneous insights can divert resources and attention away from genuine opportunities or pressing issues that require data-driven solutions.
How to Mitigate Data Hallucinations?
To minimize the risk of data hallucinations and harness the true power of data.
Practitioners must adopt a range of strategies listed below
1. Rigorous Validation
Validate data sources, assumptions, and methodologies rigorously to ensure the integrity of analyses, employing techniques such as cross-validation, sensitivity analysis, and external benchmarking.
2. Transparency and Reproducibility
Document and disclose all steps of the data analysis process to enable scrutiny and reproducibility by others, fostering accountability and facilitating peer review.
3. Diverse Perspectives
Seek input from diverse stakeholders with varied backgrounds and perspectives to challenge assumptions and mitigate biases in data interpretation and decision-making.
4. Robust Statistical Techniques
Employ robust statistical techniques and validation procedures to guard against overfitting, sampling bias, data dredging, and other potential sources of distortion.
5. Ethical Considerations
Prioritize ethical considerations in data analysis and decision-making, ensuring fairness, transparency, and accountability, while being mindful of potential unintended consequences or harmful biases.
In our data-driven age, the phenomenon of data hallucinations poses a significant challenge, threatening to undermine the reliability and credibility of analyses and decisions. By understanding their causes, implications, and mitigation strategies, we can strive to foster a data culture grounded in integrity, transparency, and rigor. Only then can we truly harness the power of data to drive meaningful insights and positive change in our increasingly complex world.
Let Etech Global Services help you make data-driven decisions with confidence. Don’t let data hallucinations lead you astray – get in touch with us to shed light on your data and illuminate the path to success.